Your CECL Implementation Roadmap
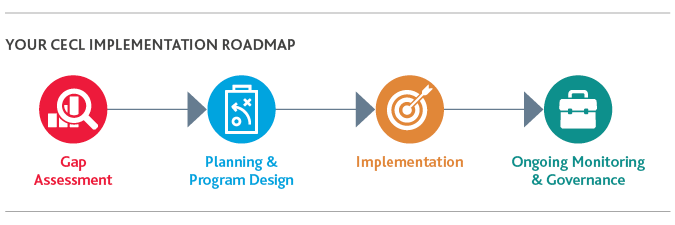
Once you have defined a target state, you can evaluate your current state to determine which existing ALLL processes you can leverage or refine and where you have holes. The goal of this gap assessment is to identify and prioritize areas for remediation and build out your detailed implementation plan.
Asset segmentation
As part of your current state analysis, you’ll want to review how your current loan portfolio is broken out for the purpose of ALLL estimations and whether its segmentation is optimal for CECL. The CECL standard calls for portfolio segments to be pooled by similar risk characteristics that most impact credit performance, and states that segmentations must be “as granular as possible while maintaining statistical significance.” Some financial institutions leverage the FDIC call code segmentation as a starting point, but it may not be granular enough or provide the right metrics to accurately reflect your portfolio.
Risk characteristics may include, but are not limited to:
- FICO ratings
- Loan-to-value ratio
- Origination exceptions
- Collateral type
- Payment structure
- Loss history
- Industry or geography of the borrower
Even if your current segmentation strategy is adequate, you must be able to prove it accurately reflects the credit risk profile of your assets with supporting documentation that shows how you made those determinations and provide evidence the segments share sufficiently similar risks.
Assessing data readiness
It cannot be overstated: data is the crux of a successful CECL implementation, and it’s where your gap assessment should begin. You will want to examine the data that your institution already has and determine what additional data you will need to effectively forecast credit risk.
Your financial institution likely already possesses much of the data it will need to comply with CECL, but it will not be enough. Under CECL, you’ll need enough data to forecast the potential losses of, for example, a 30-year mortgage on the day it is originated.
This will require a vast amount of granular, high-quality lookback or historical data, prepayment expectations, coupled with robust forward-looking economic data, that will allow you to establish trends that could point to what will happen with that mortgage over its life. Since CECL requires forecasting losses from when a loan is originated, the underlying factors driving those forecasts should be documented and tracked to avoid
material misstatements.
Data preparation alone often requires a considerable investment of time and effort that can span months. The following are four factors that will enable your institution to assess its readiness:
- Internal data completeness
- Internal data reliability
- Internal data accessibility
- Externally-sourced forecasting elements accessibility
The following internal and external data points, at a minimum, must be taken into consideration and used to support the configuration of your CECL quantitative models:
Internal data: asset segmentation, collateral type, maturity date, original balance, current balance, rate, rate type, peer data, payment type, payment frequency, cost centers, original LTV, current LTV, loan review results, call reports, FICO credit rating, region, non-performing assets, charge-off amount, recovery amount, days delinquent, disposal or recovery expenses.
External data: gross domestic product, purchase price index, home price index, money supply, consumer confidence survey, unemployment rate, consumer price index, forward looking yield curves, housing starts, peer data.
Choosing the right CECL methodology
After you have completed a data inventory and identified the gaps, you can then figure out which methodology to forecast credit losses is the best fit for your institution. CECL is principlebased, not prescriptive, which allows your financial institution to use its own judgment in developing estimation methods that best address its circumstances, and there are several methods to choose from. In fact, your institution may already be using methodologies that can help in estimating expected credit losses, such as discounted cash flows, loss rate, probability of default or provision matrix models.
Since CECL requires forecasting credit risk over the life of a loan, the new methodology you select should also assess the risks that are relevant to your specific market and take into consideration your portfolio’s composition. Before selecting a method, you should organize and understand the data needed to comply.
To choose the best methodology, a variety of models should be tested to find out which one provides the right level of granular data needed for compliance. Additionally, different estimation methods can be applied to different groups of financial assets.
To properly apply an acceptable estimation method, your institution’s credit loss estimates must be well supported.
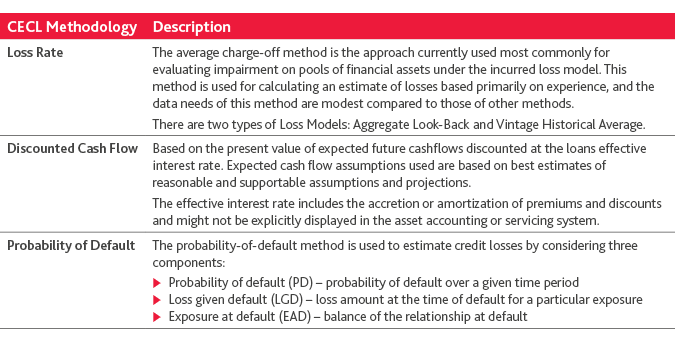
PLANNING & PROGRAM DESIGN
Determine data requirements
According to the American Bankers Association, most existing data systems only store the last 12 or 13 months of loan information, but under CECL, historical data requirements may span five years or even longer. Not only does your institution need to ensure it has enough storage space for up to a thousand times more data than it did under the incurred model, it must ensure that the data is secure and easy to access. Based on your institution’s data readiness assessment, you’ll need to update your enterprise-wide information governance program, considering the following:
- Due Diligence and Planning. Ensure that there is a comprehensive understanding of how data is used, where it’s stored and confirm that all accounting and regulatory policies are followed.
- Security, Integrity and Privacy. Protect data according to industry standards, regulations and internal requirements, while ensuring that the data is what it purports to be, and that privacy standards are maintained.
- Availability and Transparency. Ensure that data is accessible and easy to find to support business initiatives, and that it provides visibility into policies.
- Management and Enforcement. Develop policies and procedures to manage data throughout its lifetime.
- Alignment. Determine that the use of data is aligned with business functions and employs technologies that are aligned with the organization and its needs.
- Governance. Develop policies that reflect the current state of the business, but also provide flexibility to maintain updates and deliver those updates throughout the organization.
Determine data storage requirements
Integrating risk and finance data across a broad range of business functions is a major challenge for most financial institutions today. Many institutions use disparate data management systems for various types of data that provide a fragmented view and don’t account for myriad data use cases. If you lack a unified data management system, you will likely need to perform an extensive data mapping exercise.
Alternatively, your organization may decide to use CECL implementation as a trigger to kick off a larger data transformation initiative. It’s an opportunity to evaluate your current data architecture and IT systems based on the way you use information now and the way you want to use information in the future. Can you easily integrate third-party data? Can you process and contextualize data in real time? Can you easily add new
features when you add new applications or business processes?
CECL aside, these questions are critical to the continued success of your institution.
Choose the right tools
Your institution can decide between leveraging existing technologies and tools or acquiring new capabilities. There are a number of third-party software solutions designed specifically for financial institutions that integrate data from all core business functions into a single place to empower better decisions and streamline operations. It’s important to consider all interdepartmental needs when determining which vendor’s software will best position you to achieve your CECL goals.
Regardless of which tools you use, CECL success is predicated on a disciplined process, clean data and organized teams. Data should be available in usable and exportable formats, and stored in a secure database that can be updated and backed up frequently and that can be integrated into the spreadsheet environment
or a more sophisticated analytics platform. A system capable of detecting and flagging errors or stale data is ideal.
Get to know the new disclosures
There are several new disclosures that financial institutions must make under CECL. They include:
- The process by which management estimated current expected credit losses and the factors taken into consideration
- Credit quality by vintage year
- For collateral-dependent loans, the type of collateral, how the collateral secures the asset and a description of the assets secured
Examine financial reporting risks and update internal controls
Your institution also needs to plan for the potential adverse impacts CECL could have on its earnings and capital, and present that information to the board of directors and senior management. It must also consider Sarbanes-Oxley implications and develop documentation on processes and establish the necessary controls to ensure those processes are being followed correctly. Your financial institution should also consider
governance model standards included in the Federal Reserve’s SR 11-7 supervisory letter.
Also, because CECL requires losses to be forecast at loan origination, many institutions may require more time to close their books each quarter and at year-end.
Testing and execution
Estimation methods for the new accounting calculations can range anywhere from simplistic approaches to sophisticated models.Most larger institutions will use predictive models leveraging advanced data analytics. However, smaller institutions, especially those under $10 billion in assets, are weighing the costs and
benefits of a variety of CECL-compliant approaches, which fall on a spectrum between model-based and analytical.
A model-based approach leverages predictive models to forecast future borrower behavior based on statistical analysis of historical loss information. A modeled approach streamlines the reserving process and offers the most potential crossover use for risk management purposes. However, these benefits are not without
cost. The initial and ongoing investment in developing and maintaining models can be a significant barrier.
An analytical approach consists of personnel using subjective judgment to arrive at the expected credit loss based on analyses performed in spreadsheets. An analytical approach requires relatively low up-front investment and is easy to implement. However, a primarily analytical approach will resemble the
qualitative adjustment process under the current collective reserve, which many institutions consider to be onerous due to the high level of subjective judgment and manual nature of the process.
Regardless of the method used, the same objectives must be met: relevant variables need to be identified, the relationship between the variables and losses need to be estimated, and the entire end-to-end process will be subject to Sarbanes-Oxley (SOX) controls.
After performing the initial calculation, you will need to make qualitative adjustments. Modeling “what-if” scenarios with multiple data variables will take time and involve many departments. The frequency of data changes and additional inputs will also put stress on modeling and timing. Throughout the testing process, audit trails and validity of historical data must be considered and documented. Testing and documentation must
consider cross-functional interactions and feedback.
Prepare your people
CECL implementation will require disciplined change management—not just because it requires an overhaul of
accounting processes and IT systems, but because it heralds a new way of thinking. Make sure every member of the CECL engagement team understands their roles and responsibilities. Another critical piece of the people puzzle will be fostering collaboration between functional areas that may have historically operated in silos.
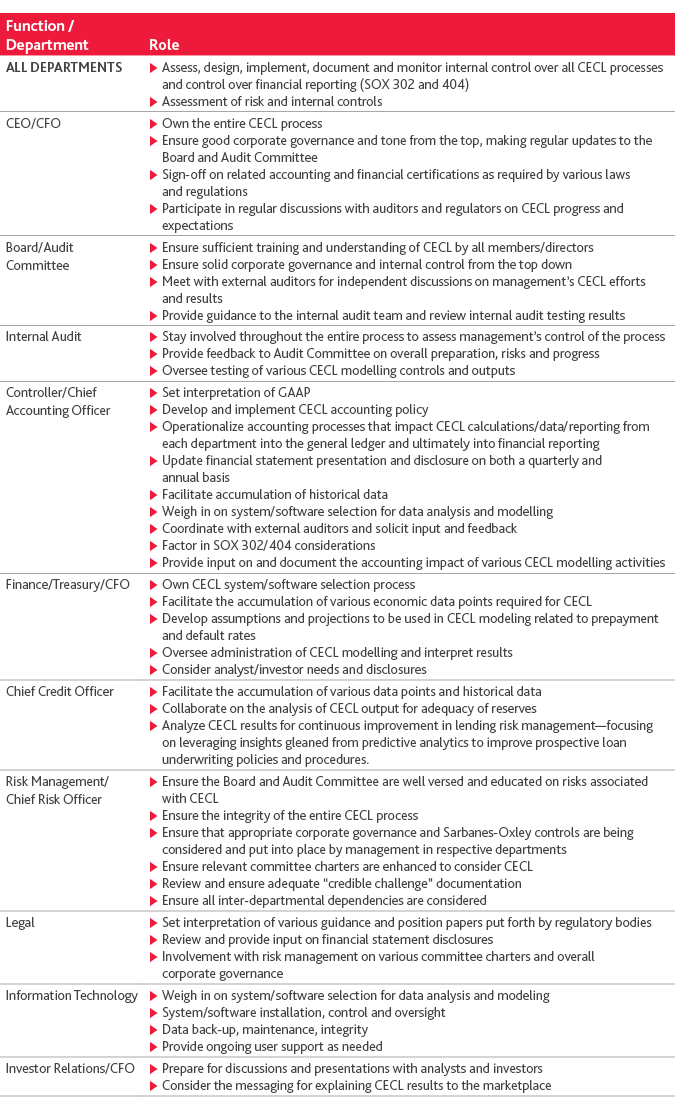
ONGOING MONITORING AND GOVERNANCE
Testing on a quarterly and annual basis is necessary to make sure your institution’s model is updated to reflect internal and external changes, such as changes in the portfolio or changes in the economy, and to identify any areas of the program that may need to be modified or require additional resources. Additional developments may require you to make adjustments to your assumptions or method of estimation.
The validity of your CECL model depends on the integrity of the underlying data. In addition to adhering to your institution’s information governance policies and protocols, you will regularly need to assess the availability and sufficiency of the data informing your CECL calculation. Like any other GAAP requirement, constant control testing and risk assessment will be necessary to ensure continued compliance long after the program has been implemented.
SHARE