Insight-Led Innovation
A Methodical Approach to Building Tomorrow Today
Digital transformation goes far beyond more efficient operations.
As middle market companies take steps towards automation, they should do so with a focus on the end game: building technical tools that will not only improve processes and cut costs, but also enable data-driven insights which will help to inform business strategies, boost revenue and increase profitability.
The evolution of a business typically follows a Darwinian path: Those that adapt to meet the changing environment survive and grow, while those that fail to evolve become extinct. But, in business, the survival of the fittest is not predetermined by natural selection—by random mutation or lucky happenstance—but rather by informed decision-making and intentional innovation guided by data-driven insight.
Disruptive technologies like artificial intelligence (AI), robotic process automation, advanced analytics, and extended reality, among others, are enabling organizations to leverage their data in new ways—beyond the primary use case—to unleash value, from new operational efficiencies to better risk management effectiveness to new sources of revenue.
But it’s disrupt or be disrupted: Competing in a digital world requires a new level of agility and insight. Relying on guesswork and gut feeling doesn’t cut it in the digital age. Organizations need to build insight engines powered by quantitative data.
Insight-led innovation fuels a culture of continuous improvement, one in which data not only augments human decision-making but also enables organizations to interact with data in entirely new ways. The concept is based on the premise that today’s innovations should pave the way for tomorrow’s, and portends a future where business intelligence guides corporate strategy, an essential determinant of risk versus return.
The Marriage of Data and Insight
Precipitating this latest era of business evolution is digital disruption and the rise of the digital economy.
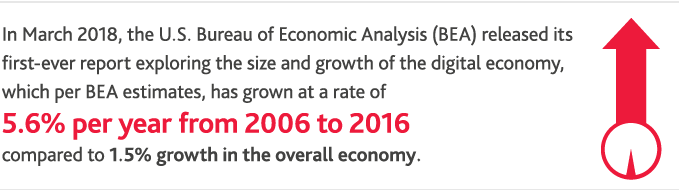
To quote our own U.S. government, “There is little doubt as to the importance of digital technology in American business and its role in fostering national economic growth and competitiveness.”
In the digital economy, data is the DNA of an organization as well as its most valuable currency. Like the sequencing of human genomes, this digital footprint tells the story of an organization’s past, present, and future, pointing the way to untapped potential and hidden value—that is, if you know how to decode it. To control their own destinies, businesses must be able to use that data to turn information into insight.
Companies that integrate data into their everyday decision-making are charting their own evolution to become insight-driven organizations, where learning is continuous and innovation is in their DNA.
These are the companies that will adapt to disruption and survive their less-nimble competitors. They are faster, smarter, and more resilient to change. Data is more accessible. Process optimization is a relentless quest. Experimentation is constant. Most importantly, their evolution is never over.
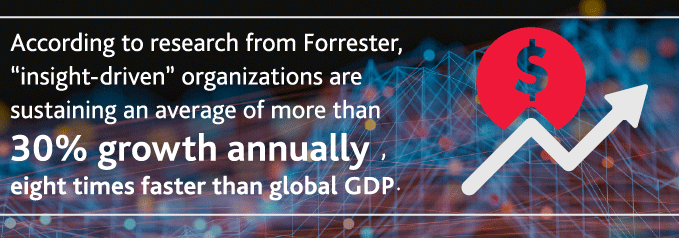
Solving for “X” While Understanding the “Why”
Most innovation is spurred by the need to provide a specific solution to an immediate, shorter-term priority—whether we’re talking automating a repetitive task to maximize operational efficiencies or migrating applications to the cloud to enable remote access. But that’s a limited view of innovation potential. “Insight-led” innovation focuses not only on solving for the immediate need, but also on maximizing the long-term potential of organizational data.
By intentionally building in data capture, every digital initiative presents an opportunity to improve and democratize business intelligence across the enterprise. Basic automation and analytics are just the beginning of this journey; insight-led innovation lays the necessary foundation for the cognitive technologies that will enable autonomous decision-making and self-optimization, ushering in the next wave of efficiency and uncovering new value.
Each innovation project, large or small, should drive incremental improvements in your organization’s ability to access, aggregate, analyze, and ultimately monetize your data.
This does not, and should not, undermine a project’s primary goal, but should ensure the intentional integration of new data sources and embedded analytics—addressing the primary need while also building in the capability upfront to capture, analyze, and leverage that data to gather valuable insights and enable more informed, data-driven, strategic business decisions down the road.
Insight-Led in Action
Imagine this scenario: A retailer with low foot traffic is looking to improve the in-store customer experience. They opt to implement mobile point of sale (mPOS) devices to attract customers to the store and speed up checkout and return lines.
The mPOS devices will likely succeed at solving the initial problem—making the checkout and return process more expeditious—but there are many other ways this purchasing data could be used. There are the existing, essential needs: Marketing needs the data to gauge the success of local ad campaigns and determine the right spend per location going forward. The accounting department needs the purchasing data to track accounts receivable, while the tax department needs both purchasing data and geographic data to calculate sales and use tax and understand the impact on their total tax liability. And don’t forget about ensuring that the way the devices collect and store cardholder data is PCI compliant. To avoid creating information silos, the retailer must ensure the data is not only compatible with existing information systems, but that it can be easily shared and accessed, as well as packaged for the benefit of multiple end users. Optimizing outcomes means optimizing crossfunctional insight from the start.
And that’s just looking at the basic reporting needs. The mPOS purchasing data is a potential customer intelligence goldmine—especially in aggregate with data from other POS systems. The retailer can leverage insights on buyer behavior for demand sensing or incorporate it into a customer journey map to enable personalization at scale. Embedding analytics at the application level can reveal patterns and trends in product, staff, and store performance that further optimize the purchase process. Real-time data streams can also be connected to the inventory management system to keep on top of stock levels and reduce errors from manual data entry. The original intent might be reducing in-store points of friction, but applying an insight-led lens exponentially increases the ROI.
“Dark” data essentially equals no data
As big data gets bigger, converting data to actionable insight is easier said than done. Data might be the new oil, but in the words of Ginni Rometty, CEO of IBM, “Value goes to those who refine it, not to those who just hold it.”
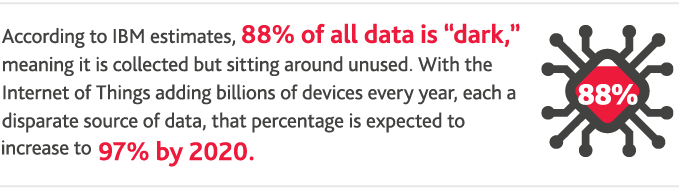
The amount of lost value in this dark data can’t be quantified— but for some businesses, it could be the difference between survival and extinction.
There is a reason the vast majority of enterprise data is “dark”—acquiring data is rarely the issue, but rather figuring out how to wrangle it into some semblance of order. In an era of smartphones and the Internet of Things, not only is data proliferating at exponential rates, it’s often disparate—housed in many different locations—and increasingly unstructured, meaning the information format doesn’t fit within conventional databases.
Between the complexity of emerging data types, the siloed ways in which it is housed, and the sheer volume, manual methods of data management and analysis simply can’t keep up.
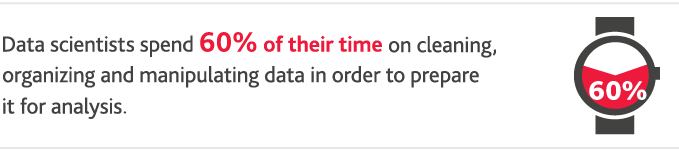
This, in and of itself, is a case for insight-led innovation. If organizations can’t find the data they need—or if they don’t know what relevant data they have to begin with—not only can it not be used for its primary purpose, but all of the value that lies in that data will be rendered just as useless.
Bringing together disparate structured data sets can address some of the dark data problem. Just think about the sheer volume of financial and operational information your annual audit requires. From the general ledger (and all the supporting source documents) to contracts and loan statements to payroll reports and board minutes, the audit is a veritable treasure trove of structured data with untapped value. If all that data is centralized in one place, data mining and advanced analytics techniques can be applied to reveal additional value. Not only can extracted insights point to current weaknesses in internal controls and areas of risk, they can be used to model future risk and economic scenarios and augment decision-making through competitive benchmarking.
Insight-Led in Action
Picture this: The finance department of a medical equipment manufacturer is drowning in work—and underperforming as a result. To free up time and resources, the company focuses on eliminating excessive manual work, starting with a simple, lowcost solution to an excessively manual but necessary task: automating slide creation for the monthly CFO update. They plan to deploy a script-driven “bot” to automatically extract and populate the information for the presentation slides, including all the formatting changes and updates in the notes. Once the data is consolidated into a unified database, the manufacturer will also be able to add value by analyzing trends over time.
The current approach involves pulling data from multiple Excel files, followed by manual data entry and manipulation. To automate presentation development, the manufacturer needs to first aggregate the information required for the monthly update into a single database. How much work is necessary to combine these disparate data sets is dependent on the heterogeneity and quality of data input, and their approach to data integration.
A one-time data migration, for example, in which data is simply moved from one location to another, offers a quick fix, saving the department a significant amount of time each quarter—increasing productivity and accuracy, and reducing cost. Longer-term, however, the finance department would save even more time and money through automated data synchronization.
By innovating with insight and considering different methods of data synchronization at the outset, the company would ideally give thought to their current needs, but to future use cases for this information and data capture across the enterprise. A standalone robotic process automation project is relatively easy, but the company wants to eventually eliminate all repetitive manual activities. This bot is likely the first of many—so what can be done now to capture necessary data and configure processes that would enable incremental automation and also provide additional business insights? Can the bot feed into a visual dashboard that continuously tracks these financial reporting KPIs, drills down into the underlying metrics, and displays them in an easy-to-read format? Should that dashboard be cloud-based to give anywhere access to other key business stakeholders? What information could be captured now that would be helpful in informing strategic business decisions?
What starts as a simple need and a quick win can ultimately trigger the transformation of financial reporting from once-a-month to real-time, and result in deeper business insights with wider accessibility.
Building Insight into Innovation
Transforming information into insight starts with reorganizing your data to streamline the insight discovery process. Ungoverned data requires significantly more time to prepare for analysis. A complete information governance overhaul may not be realistic all in one shot—which is where insight-led innovation comes into play. With proper planning at the outset, every digital initiative is an opportunity to drive incremental improvement in data management and provisioning, integrate multiple sources of data, and harness new insight and value.
Imagine, for example, a relatively contained finance initiative to track and visualize key financial metrics in a centralized dashboard that updates in real-time. The initiative could stop there, as a simple, efficient reporting tool—or the dashboard could be made actionable by triggering automatic alerts when numbers are above or below a pre-determined threshold, or flag significant variances from the budget. Customizable views could be created to more effectively communicate with different company stakeholders with different needs and different ways of interpreting information. Additional analytical capabilities could be built in to show historical trends, identify potential business issues, and forecast future scenarios. What started as a financial reporting mechanism can be transformed into a system of insight.
Any digital initiative must not only take into consideration the way you use information now but the way you want to use information in the future. Many organizations are still in the process of digitizing analog information and tasks, with a focus on the automation of routine business processes. That does not mean, however, that insight-led innovation is premature. Organizations would be wise to start good data management habits now, being mindful of the value they hope to derive from analytics in the future.
Eventually, every business process, from core operational processes like customer acquisition to post-purchase support, to management processes like budgeting and risk management to support processes like HR and accounting, should be data-driven, with analytics embedded throughout. The journey to operationalizing analytics across the enterprise may start small, with ad-hoc adoption of analytics in dashboards and reporting, paving the way for more sophisticated analytics tools and business intelligence down the road.
It’s perfectly okay for analytics to exist in pockets—as long as you continue to think through and discover upfront what data should be captured and what insights would be most valuable for determining strategic direction, applying a crossfunctional lens. Learn and understand what you’d like to know and why, then explore ways to build it in. Communicate progress and coordinate plans at the enterprise level to prevent bottlenecks and scale faster.
Most of these early analytics projects will likely be based on structured data tied to specific operational functions for clearly defined tasks. In other words, you already know what you want to use the data for at the onset. But how do you find new insights in data when you don’t know what you’re looking for? Entirely new revenue streams don’t just jump out from data ordered in neat columns and rows. To maximize the full value of big data, you need to be able to extract insights from both structured and unstructured data, in combinations that are seldom predefined. More sophisticated analytics and machine learning initiatives will require a cohesive analytics architecture that integrates both data and analytic applications.
Insight-Led to Become Insight-Driven
Insight-driven organizations are made, not born. The power of data cannot be harnessed without strategic intent and foresight. Data is fuel, but insights are the engine. And even insight is only as useful as it is actionable. The journey toward “insight-driven” starts with insight-led innovation: focused on improving insight collection and capabilities at every opportunity. As technological disruption continues to accelerate the pace of change, the ability to turn data into insight and insight into action may be the evolutionary trait that determines fitness for survival.
SHARE