Gaining User Adoption in Data Analytics Projects (Part 2)
Gaining User Adoption in Data Analytics Projects (Part 2)
In my last blog post (Gaining User Adoption in Data Analytics Projects - Part 1), I discussed the critical role user adoption plays in becoming a data-driven organization. In this blog, we’ll identify key strategies to incorporate at the start of your data analytics project to gain greater user adoption and realize the full potential of your data.
When to Start Building User Adoption
User adoption doesn’t start with an email announcement and some basic training materials when the project is winding down; it starts from the very beginning! IT should be fully engaged with the business throughout the entire lifecycle of the project. Not only does this ensure the end product provides the outcomes the users expect, but it also helps build a greater sense of shared ownership in its success.
Four Key Strategies to Include at the Start of Your Data Analytics Project
Some key strategies that I recommend you start incorporating at the start of the project include:
1) Define a team of business intelligence (BI) Champions to see the project through
The BI team should include individuals who will ultimately rely on the solution to achieve better business results. This team will own, define, and govern designs and calculations. They will be responsible for a number of tasks, including:
- Identifying expected results, which are used during the development and testing process to confirm report outputs
- Setting expectations that this will be a critical element to the project’s success
- Making sure all decisions are documented for future reference by both the IT team as well as others in the organization
2) Identify and prioritize requirements
Working with the BI champions, you can begin to identify as many features as possible. But don’t get bogged down with the pressure of needing to figure out everything at the beginning. Prioritize the features and determine which ones will provide the most business value. This is where you’ll want to start.
3) Set some schedule or cadence for the delivery of prioritized features
I suggest an iterative delivery of BI projects for the following reasons:
- BI projects tend to be more nebulous to business users and they might have difficulty in visualizing the solution. Using a tool like Power BI is a great way to create mockup visualizations using sample data sets and validate certain calculations.
- Using an agile approach will allow you to quickly deliver a portion of the solution to the business, and get their feedback before moving forward.
- Based on their feedback, you can make adjustments and continue the refine and review your progress in cycles. Not only does the business feel engaged, but this also allows them to learn about the process and some of the potential capabilities along the way.
- By delivering on the highest impact features first, as quickly as possible, you’ll be able to show immediate results and earn the business’ trust.
The business now sees the potential of the process and is excited to maintain momentum. They want to start working on the next set of features!
4) Confirm the validity and quality of the source data
This could require a separate engagement on its own, especially if you identify data quality issues. However, if the source data is inconsistent, you’ll never be able to produce solid and consistent results, and your solution will be doomed from the start. Create a data governance team to define and implement data policies and best practices. Establish methodologies to audit new data as it is entered into the system.
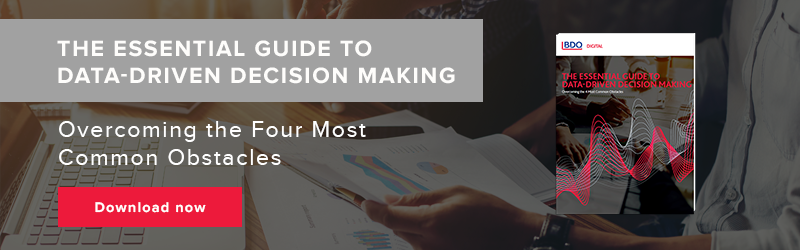
SHARE